RESEARCH DATA
Data are an essential component of empirical research. Modern technology allows the creation of much larger volumes of data than in the past, and many new tools are emerging to analyse, store, manage, and share data.
Scientific data are any information that can be gathered through observation, experimentation, surveys, or other means, the analysis of which is intended to confirm (or refute) the hypothesis of the research being conducted.
With the development and application of Open Science in practise, projects are increasingly required to open/publish not only the results of the research carried out, but also the data generated to produce them in order to receive funding. To this end, data generated during a research project must be made openly available to the public, through open-access data repositories or through publication in data journals. Data opened in this way can be reused by other researchers. Alternatively, data can be cited separately, giving the researcher the added benefit of visibility and influence.
Scientific data are any information that can be gathered through observation, experimentation, surveys, or other means, the analysis of which is intended to confirm (or refute) the hypothesis of the research being conducted.
With the development and application of Open Science in practise, projects are increasingly required to open/publish not only the results of the research carried out, but also the data generated to produce them in order to receive funding. To this end, data generated during a research project must be made openly available to the public, through open-access data repositories or through publication in data journals. Data opened in this way can be reused by other researchers. Alternatively, data can be cited separately, giving the researcher the added benefit of visibility and influence.
Data types
Scientific data includes not only the results of experiments but also primary and intermediate data (such as spreadsheets, laboratory notes, diaries, questionnaires, transcripts, codes, audio recordings, video recordings, photographs, test answers, slides, artefacts, samples, collections of digital objects, data files, databases, algorithms, research methodologies, workflows, software content (input/output data, schematics, data files), protocols, etc.). However, depending on the research discipline and context, different institutions may distinguish different types of data:
NSF (National Science Foundation) distinguished data types:
- Data (numerical or qualitative)
- Publications
- Samples (e.g. blood, soil etc.)
- Physical collections (e.g. herbarium, archeological finds etc.)
- Software
- Research models
NEH (National Endowment for the Humanities) distinguished data types:
- Citations
- Software codes
- Algorithms
- Digital tools
- Geospatial coordinates
- Documentation
- Reports
- Articles
In order for data to be findable, it must be described with a metadata description. The components of the data description (metadata) are similar to the ones of the bibliographic description of research publications.
Metadata components for describing data:
Data description (metadata) are compiled according to certain metadata standards:
*DOI (Digital Object Identifier) – is a global, unique, and persistent digital name of an object. It does not change over time despite the possible changes in the object’s location, and is intended for an accurate identification of an online published object in the internet space.
Metadata components for describing data:
- Author(s) – name(s) of the data creator(s) (individuals or organizational unites) name(s).
- Publication date – indicate the year in which the data was published/placed in the repository.
- Name – the full name of the dataset, including the version number (if applicable).
- Source / Publisher – indicate where the data were published (repository or data journal).
- Link / Identifier – a link (URL) for direct access to the data on the Internet or a unique, permanent identifier (e.g., DOI*).
Data description (metadata) are compiled according to certain metadata standards:
- CERIF (Common European Research Information Format) – a common standard for the description of metadata in various disciplines for the European Union countries.
- Dublin Core (Dublin Core Metadata) – a standard for describing metadata for various disciplines.
- DDI (Document, Discover and Interoperate) – an international standard for the description of survey data in social, behavioral, economic and health sciences.
- DICOM (Digital Imaging and Communications in Medicine) – a standard for describing biomedical data.
- CIF (Crystallographic Information File) – a metadata standard for describing data from crystallographic and other structural studies.
*DOI (Digital Object Identifier) – is a global, unique, and persistent digital name of an object. It does not change over time despite the possible changes in the object’s location, and is intended for an accurate identification of an online published object in the internet space.
Background data are needed to understand, correctly interpret and be able to reuse the data for secondary analysis.
Background data can be considered:
Background data can be considered:
- code descriptions
- questionnaires
- description of methodologies
- reports
- conference poster presentations
- articles
- information on websites, blogs, etc.
Research Data Management
In order to be published, the data must be properly collected, presented, described, and hosted throughout the study. Proper collection, preparation, and publication of scientific data are collectively referred to as Scientific Data Management (SDM).
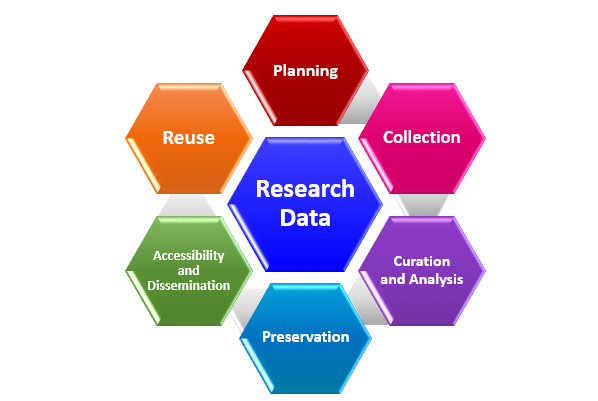
Effective RDM must be performed at all stages of the cycle.
Planning stage involves:
- Initial decision on whether new data will be collected, or already existing data sets will be used in the study
- Selection of data repository
- Selection of data formats and metadata standards
- Identification of confidentiality, privacy and other ethical issues
- Identification of potential data users
- Assessment of the possible costs surrounding data management
- Determination of the procedures needed for file organizing, backup creation and data storing
- Creation of quality assurance protocols
- Establishing data security measures and setting access restrictions
Primary data may need to be refined, standardized or otherwise processed for enabling their further analysis. Therefore, at this stage it is particularly important that all manipulations made to the primary data are documented. It is essential to describe all used analysis procedures, models, as well as the specifications of hardware and software.
Data preservation stage:
Depending on the requirements of the selected repository and / or funder the format of data that will be made public is determined at this stage, as well as proceeding with the further data curation / cleaning (e.g. depersonalization) and documentation. All documentation describing the data must be reviewed to ensure that it is comprehensive enough to enable the discovery and reuse of the published data.
Data Accessibility and Dissemination stage:
The processed and described data are published in scientific articles, data journals, reports, and together with additional information are stored in data repositories or archives.
Who is involved in the RDM process?
Publishers and journals publish research results and scientific discoveries based on them. Publishers and editorial boards of journals are increasingly encouraging authors to cite data (both self-produced and external that have been reused in the study). Some journals, e.g., PLOS (Public Library of Sciences) include the requirement that the data used in research must be published in data repositories.
Due to the large number of stakeholders involved in the RDM process, effective RDM requires close cooperation between all participants in the process.
Scientists and researchers, data creators, and users. They plan the studies, anticipate what data will be collected, collect and process the data, as well as determine how the data will be analysed and what possible conclusions they may provide.
Universities and research institutes – set internal RDM policies. They can provide the necessary resources required for RDM implementation in practise, such as RDM training, support in developing RDM plans, hardware and/or software, and related consulting (IT departments), as well as data archiving services (institutional repositories).
Data repositories – supervises the data by ensuring their long-term preservation and access. Data repositories work with data creators to ensure the long-term usefulness of the data and impose necessary access restrictions (e.g., embargo periods or other access restrictions related to the requirements of the institution or the funder), and ensure data security and respect for intellectual property rights.
Users – representatives of various fields using the published data. Data re-users can be: data producers themselves and other researchers, who examine the data from other aspects than in the original research, compare similar data obtained at later stages, and/or seek to verify the reliability of the research results; teachers who use the data for teaching purposes; students, employing the data for preparation of their graduation works; business, political or private sector representatives whose decisions are often based on the data; journalists aiming to make their published information more reliable; and interested members of the general society.
Funders provide the necessary resources for the research. Today, increasingly funding institutions are demanding an RDM plan to increase transparency in the execution of their funded projects. By encouraging the re-use of data collected during the project, funders are also seeking to increase their return on investment.
Publishers and journals publish research results and scientific discoveries based on them. Publishers and editorial boards of journals are increasingly encouraging authors to cite data (both self-produced and external that have been reused in the study). Some journals, e.g., PLOS (Public Library of Sciences) include the requirement that the data used in research must be published in data repositories.
Due to the large number of stakeholders involved in the RDM process, effective RDM requires close cooperation between all participants in the process.
Why is data management important?
RDM helps researchers organise research more efficiently:
RDM ensures that the data will be preserved and made available for future research, interpretation, and reuse:
RDM helps researchers organise research more efficiently:
- In the case of a large project that generates different types of data, even researchers themselves may get lost in the variety and abundance of data. RDM helps optimise the use of data in the active research phase.
- Often data collection, processing, or analysis tasks are performed by graduate students or other project participants (staff members or even representatives of other departments or institutions). Therefore, RDM helps to cooperate with other research participants and facilitates knowledge transfer in the context of staff changes.
RDM ensures that the data will be preserved and made available for future research, interpretation, and reuse:
- At the end of a research or project, the data can be used to answer additional questions that were not addressed in the original research.
- Furthermore, if a similar research is to be carried out in the future, the data collected from the original research can be easily used to compare the results, which would be practically impossible if the data were not processed systematically.
- RDM can also increase the transparency of research and the reliability of data, as properly prepared data allow them to be used to validate the results of research.
- Properly collected and described data can be discovered and reused by third parties (users), thus ensuring not only a higher return on investment in projects due to the reuse of data for innovation and progress but also to promote the development of citizen science and provides the possibility to employ data for study and teaching purposes.
Documents regulating research data management in Lithuania
Research Data Management (RDM) principles in Europe are based on the European Commission‘s (EC) 2017 March 17 Guidelines on the Rules for Open Access to Scientific Publications and Open Access to Research Data in Horizon 2020. More information on the European Commission‘s open science and research data management policies can be found on the OpenAIRE website.
The principles of data management of the projects financed by the Research Council of Lithuania (RCL) are defined in 2016. February 29 by resolution no. VIII-2 Guidelines for Open Access to Scientific Publications and Data.
The key provisions in the EC and RCL guidelines are similar and emphasize:
At VILNIUS TECH research data management is carried out in accordance with „Guidelines for Open Access to Data and Scientific Publication of Vilnius Gediminas Technical University“ approved by the Rector‘s Order No. 1231 on 2016 December 6.
Highlights:
FAIR principles
All regulations require that research data would be collected, formatted, described, and stored in accordance with FAIR principles (A set of guiding principles to make data Findable, Accessible, Interoperable, and Reusable).
The principles of data management of the projects financed by the Research Council of Lithuania (RCL) are defined in 2016. February 29 by resolution no. VIII-2 Guidelines for Open Access to Scientific Publications and Data.
The key provisions in the EC and RCL guidelines are similar and emphasize:
- the importance of presenting a research data management plan;
- data storage in research data repositories;
- ensuring open access to data through open content licenses;
- ensuring long-term data preservation after the end of the project;
- a comprehensive description of the data, providing sufficient information and tools to verify the research results;
- linking data to relevant publications.
At VILNIUS TECH research data management is carried out in accordance with „Guidelines for Open Access to Data and Scientific Publication of Vilnius Gediminas Technical University“ approved by the Rector‘s Order No. 1231 on 2016 December 6.
Highlights:
- research data obtained from publicly funded project activities must be kept upon open access according to the requirements of the funding institution;
- at the end of the project, data storage and submission to an open-access repository must be ensured;
- ensure timely open access to data and their metadata, which should coincide with the announcement of the relevant publication.
FAIR principles
All regulations require that research data would be collected, formatted, described, and stored in accordance with FAIR principles (A set of guiding principles to make data Findable, Accessible, Interoperable, and Reusable).
Data Management Plans (DMPs)
Increasingly, applications for project funding are required to be accompanied by a research data management plan.
A Research Data Management Plan (DMP) is a formal document that describes the processes that will be performed on the data generated or reused in a study, identifying the specific tools and strategies that will be used at all stages of the data management cycle.
A Research Data Management Plan (DMP) is a formal document that describes the processes that will be performed on the data generated or reused in a study, identifying the specific tools and strategies that will be used at all stages of the data management cycle.
The DMP should detail all key aspects of data management throughout the life cycle of the research. The DMPs follow a common structure, answering several key questions:
- What data will be created, generated, and collected?
- In what format will the data be collected and what is the expected volume?
- How will the data be managed during and after the project?
- What methods and metadata standards will be used? (Different metadata standards are used in different scientific fields/disciplines and need to be foreseen prior to the start of data collection)
- How will long-term data storage be ensured: in which repository will the data be hosted, and how much will it cost?
- What access to the data is envisaged: will it be open access and how will the data be shared?
The Digital Curation Centre provides a checklist of questions to be answered in the preparation of the DMP (Digital Curation Centre’s Checklist for a Data Management Plan):
What data will be collected or created?
A description of the data is required, which describes the type, format, and scope of the data, together with a justification for the choice of a particular format. If there are any storage implications due to the format or volume of the data, they should be included as well.
How will the data be collected or created?
A description of the data collection methods to be used, including the standards that dictate these methods.
It is also necessary to anticipate how data files will be handled, including the management of different versions, and what quality assurance protocols will be implemented during and after data collection and processing.
What metadata and supporting documentation will be provided to the data?
A proper description of the data set (metadata) is crucial to ensure that the data are discoverable.
It is important to identify what information will be needed to make the data understandable to future secondary users of the data or to the data creators themselves. In other words, it is necessary to describe the additional data that will need to be provided to the data set to be interpreted and used in the future.
Additional data may include descriptions of methodologies, code descriptions containing definitions of variables and their values, questionnaires, descriptions of hardware or software, the analytical procedures used and their conditions, and more.
How will ethical issues be addressed?
The DMP should show that you have considered key issues related to laws and guidelines for the protection of human subjects. If the project conducts research involving human subjects, provisions for protecting their confidentiality should be described. This should include strategies for handling and storing sensitive data, restricting access to sensitive data, or depersonalising data to make them suitable for publication.
How will copyright and intellectual property rights issues be handled?
This is where legal issues of data ownership need to be addressed. The owner of the data and the conditions of use should be identified. If third-party data are reused, especially if it is protected by proprietary rights, permissions to use the data must be obtained and provided by the data creator.
How will the data be stored and backed up during the research?
A description of the data retention provisions and the degree to which they apply to the type and scope of your data is required. Storage provisions should include systematic backup plans for data files.
How will access and data security be managed?
If the data are sensitive or should be otherwise restricted, with access limited to Authorised Project Participants only, it is necessary to describe in detail the security measures that will be applied to protect the data. It is also necessary to specify the standards to which these security measures will be applied.
What data should be stored, published, and/or archived?
Considering how the data might be used in the future by others or by the data creators themselves, it is necessary to consider the potential value of the data and the effort and resources that will be needed to prepare the data sets for preservation and long-term access.
What is the long-term preservation plan for the dataset?
It is necessary to choose a repository (data storage) that will be used to archive the data, ensuring that it will be preserved and available for use in the near future and in the long term.
How will the data be shared?
The mechanism that will be used to share the data must be defined. It needs to describe how the data will be available to other interested users and how the data files will be made available to them. The preferences of how the data should be acknowledged or cited by other users should also be specified.
Are restrictions on data sharing necessary?
If privacy concerns affect the ability to share project data, it is necessary to consider how these concerns can be addressed so that the data can be made publicly available (through the provision of de-personalised versions of the data, by requiring a data use agreement, or through other mechanisms). Alternatively, it may be possible to opt for an embargo on the data, restricting access to authorised users (project participants) for a certain period. In this case, the reasons for the embargo should be explained.
Who will be responsible for data management?
If possible, name the specific person who will be responsible for implementing the data management plan.
What resources will be needed to implement the DMP?
For some projects based on complex data, due to the type of data, the size of the data, or the fact that the project activities are geographically distributed across different institutions, data management may require specialised expertise or equipment.
It is also necessary to consider the costs associated with the performance of ongoing data management tasks and the long-term preservation of data in the repository.
Answering these questions will not only ensure compliance with data governance requirements but will also help to better prepare the implementation of data governance strategies.
What data will be collected or created?
A description of the data is required, which describes the type, format, and scope of the data, together with a justification for the choice of a particular format. If there are any storage implications due to the format or volume of the data, they should be included as well.
How will the data be collected or created?
A description of the data collection methods to be used, including the standards that dictate these methods.
It is also necessary to anticipate how data files will be handled, including the management of different versions, and what quality assurance protocols will be implemented during and after data collection and processing.
What metadata and supporting documentation will be provided to the data?
A proper description of the data set (metadata) is crucial to ensure that the data are discoverable.
It is important to identify what information will be needed to make the data understandable to future secondary users of the data or to the data creators themselves. In other words, it is necessary to describe the additional data that will need to be provided to the data set to be interpreted and used in the future.
Additional data may include descriptions of methodologies, code descriptions containing definitions of variables and their values, questionnaires, descriptions of hardware or software, the analytical procedures used and their conditions, and more.
How will ethical issues be addressed?
The DMP should show that you have considered key issues related to laws and guidelines for the protection of human subjects. If the project conducts research involving human subjects, provisions for protecting their confidentiality should be described. This should include strategies for handling and storing sensitive data, restricting access to sensitive data, or depersonalising data to make them suitable for publication.
How will copyright and intellectual property rights issues be handled?
This is where legal issues of data ownership need to be addressed. The owner of the data and the conditions of use should be identified. If third-party data are reused, especially if it is protected by proprietary rights, permissions to use the data must be obtained and provided by the data creator.
How will the data be stored and backed up during the research?
A description of the data retention provisions and the degree to which they apply to the type and scope of your data is required. Storage provisions should include systematic backup plans for data files.
How will access and data security be managed?
If the data are sensitive or should be otherwise restricted, with access limited to Authorised Project Participants only, it is necessary to describe in detail the security measures that will be applied to protect the data. It is also necessary to specify the standards to which these security measures will be applied.
What data should be stored, published, and/or archived?
Considering how the data might be used in the future by others or by the data creators themselves, it is necessary to consider the potential value of the data and the effort and resources that will be needed to prepare the data sets for preservation and long-term access.
What is the long-term preservation plan for the dataset?
It is necessary to choose a repository (data storage) that will be used to archive the data, ensuring that it will be preserved and available for use in the near future and in the long term.
How will the data be shared?
The mechanism that will be used to share the data must be defined. It needs to describe how the data will be available to other interested users and how the data files will be made available to them. The preferences of how the data should be acknowledged or cited by other users should also be specified.
Are restrictions on data sharing necessary?
If privacy concerns affect the ability to share project data, it is necessary to consider how these concerns can be addressed so that the data can be made publicly available (through the provision of de-personalised versions of the data, by requiring a data use agreement, or through other mechanisms). Alternatively, it may be possible to opt for an embargo on the data, restricting access to authorised users (project participants) for a certain period. In this case, the reasons for the embargo should be explained.
Who will be responsible for data management?
If possible, name the specific person who will be responsible for implementing the data management plan.
What resources will be needed to implement the DMP?
For some projects based on complex data, due to the type of data, the size of the data, or the fact that the project activities are geographically distributed across different institutions, data management may require specialised expertise or equipment.
It is also necessary to consider the costs associated with the performance of ongoing data management tasks and the long-term preservation of data in the repository.
Answering these questions will not only ensure compliance with data governance requirements but will also help to better prepare the implementation of data governance strategies.
There are several free online tools for creating data management plans. The most widely used tools are DMPTool and DMPOnline, developed by the University of California Curatorial Center of the California Digital Library and the UK Digital Curation Center (DCC).
Both tools are designed to facilitate the preparation of DMPs. They provide a step-by-step guide that allows researchers to create customised data management plans. Guidance and templates are provided for researchers to meet the requirements of different funding agencies. As funding agency policies change, the information in the templates provided is updated. But it is also possible to develop non-funder-specific plans, following the overall structure of the DMP. Institutions can also adapt the tools to their individual needs.
For Lithuanian researchers, the preparation of DMP is relevant for applications to Europe-Horizon and RCL-funded projects:
Both tools are designed to facilitate the preparation of DMPs. They provide a step-by-step guide that allows researchers to create customised data management plans. Guidance and templates are provided for researchers to meet the requirements of different funding agencies. As funding agency policies change, the information in the templates provided is updated. But it is also possible to develop non-funder-specific plans, following the overall structure of the DMP. Institutions can also adapt the tools to their individual needs.
For Lithuanian researchers, the preparation of DMP is relevant for applications to Europe-Horizon and RCL-funded projects:
- In the DMPOnline platform, there is a publicly available DMP template developed specifically in accordance with Europe-Horizon projects’ requirements.
- Detailed RCL requirements for DVPs are listed here (available only in Lithuanian) >>>
Research data collection and storage
The suitability of the format for the data is dependent on the type of data itself and the specifics of its generation, as well as the equipment used. Furthermore, when preparing data for publication in data repositories or data journals, the formats supported by the repositories and recommended by the publishers must be considered as they can vary. However, it is recommended that, where possible, the choice of the data format should consider several key criteria.
Recommended data formats:
- Is the data format widely used?
- Is the format suitable for long-term storage?
- Is the format open, and does not require licenced software to use it?
- What is the complexity of the format? It is recommended to choose simpler formats?
- Can compression (archiving) be applied to the format and is not detrimental to the data quality?
Recommended data formats:
Data type | Recommended formats |
Text | PDF (the most appropriate: PDF/A) without formatting: TXT can be edited: ODT, RTF, HTML for text with formulas: LaTeX (TEX) |
Tables | CSV / TSV Numerical data: HDF5 |
Graphics | Raster: PNG, TIFF Vector: SVG, EPS |
Multimedia | Multimedia: MKV, WebM, Video: AV1, VP9 Audio: FLAC, WAV, Vorbis, Opus |
Linked and/or structured data | SIARD, Dump, XML, CSV / TSV, HDF5, JSON, YAML |
Data Repositories
These registries can be used both to find the most suitable repository for data storage, and for searching of existing data sets.
re3data – the Registry of Research Data Repositories – is the most widely used search engine for research data repositories. It helps to find discipline specific repositories among more than 2000 registered ones. You can search by topic, country, content type, and more. This search engine is a service of DataCite (a non-profit organization that provides persistent digital identifiers (DOI) for research data).
Other data repository registries:
re3data – the Registry of Research Data Repositories – is the most widely used search engine for research data repositories. It helps to find discipline specific repositories among more than 2000 registered ones. You can search by topic, country, content type, and more. This search engine is a service of DataCite (a non-profit organization that provides persistent digital identifiers (DOI) for research data).
Other data repository registries:
- FAIRsharing (databases catalogue)
- Open Access Infrastructure for Research in Europe (OpenAIRE / Explore)
- Directory of Open Access Repositories (OpenDOAR)
- Master Data Repository List (Clarivate Analytics)
These repositories accumulates the research data and/or results of all disciplines. Data deposition, archiving and access are free. Each data set receives a permanent digital identifier DOI.
- Zenodo – this repository is linked to Horizon 2020 and OpenAIRE projects. The repository is funded by the European Commission.
- DRYAD
- FigShare
- 4TU.ResearchData
- B2SHARE
- Mendeley.Data
Data journals
The number of journals dedicated for publishing data is growing rapidly. The most popular data journals are listed below.
- Biodiversity Data Journal (Pensoft)
- Biomedical Data Journal (Procon)
- BMC Research Notes (Springer Nature)
- Data (MDPI)
- Data in Brief (Elsevier)
- Earth System Science Data (Copernicus Publications)
- Ecology (Wiley)
- Geoscience Data Journal (Wiley)
- F1000Research (Taylor & Francis Group)
- Genomics Data (Elsevier)
- Geoscience Data Journal (Wiley)
- Geoscientific Model Development (EGU Publications)
- GigaScience (Oxford Academic)
- International Journal of Robotics Research (SAGE Journals)
- Journal of Chemical and Engineering Data (ACS Publications)
- Journal of Physical and Chemical Reference Data (AIP Publishing)
- Nuclear Data Sheets (Elsevier)
- Research Data Journal for the Humanities and Social Sciences (Brill)
- Scientific Data (Springer Nature)
Search and citation of research data
For research papers, coursework, theses, and dissertations, you can reuse existing published data instead of collecting or creating it yourself, saving you both time and resources. As a result, researchers, government organisations, and other institutions are increasingly making the data sets they create available to other researchers for reuse.
Research data for your research can be found in the same data repositories and data journals where you can host and publish your research data. Each data repository has different search methods. Therefore, for each data repository, we recommend that you consult the repository's user guide on the repository's website, which will help you to optimise your search and efficiently find the datasets you need.
To facilitate your search, additional tools have been developed to help you search for data relevant to your research topic in several data repositories at the same time, such as:
Research data for your research can be found in the same data repositories and data journals where you can host and publish your research data. Each data repository has different search methods. Therefore, for each data repository, we recommend that you consult the repository's user guide on the repository's website, which will help you to optimise your search and efficiently find the datasets you need.
To facilitate your search, additional tools have been developed to help you search for data relevant to your research topic in several data repositories at the same time, such as:
- DataCite – helps to find the required data sets in several repositories according to the topic you are interested in by using Datacite search.
- Mendeley.Data – is a research data search engine where researchers can search within > 25 million datasets in both thematic and multidisciplinary data repositories.
- Data Observation Network for Earth (DataONE) – provides capabilities of data search for Earth observation research data across a wide network of subject related repositories.
- CESSDA Data Catalogue – allows search of social sciences datasets in the repositories of the European Consortium for Social Sciences Data Archives.
Research data is also a scientific output. Therefore, when using data that are not of your creation within your work, it must be cited like any other source of information, and the data used must be cited in the text and included in the reference list. The citation and bibliographic description of the data shall be constructed in the same way as for scientific publications, indicating the main components of the metadata.
The general structure of the bibliographic description of data:
Author(s). (Year of publication). Title. Version. Publisher. Type of source. Identifier.
As with publication citations, the structure of the bibliographic record depends on the citation style used and, therefore, the order and formatting of the individual components in bibliographic descriptions may vary.
The general structure of the bibliographic description of data:
Author(s). (Year of publication). Title. Version. Publisher. Type of source. Identifier.
As with publication citations, the structure of the bibliographic record depends on the citation style used and, therefore, the order and formatting of the individual components in bibliographic descriptions may vary.
Additional information resources
- FOSTER is an organization that provides comprehensive information on open science in order to fill existing knowledge gaps in the academic community. On scientific data management topics, the organization provides training materials, as well as free courses (Open and FAIR Research Data and Managing and Sharing Research Data), and provides a link to the Open Science Training Handbook on a variety of open science topics, including open research data.
- OpenAIRE – is an organization funded by the European Commission that is actively involved in shaping open science policy. The organization also provides comprehensive information and guides on scientific data management and other open science topics. Summarized information on research data management is provided in the A Research Data Management Handbook.
- Open Knowledge Foundation – it is a not-for-profit organization. Their mission is “to create a more open world – a world where all non-personal information is open, free for everyone to use, build on and share; and creators and innovators are fairly recognized and rewarded”. They have developed an Open data handbook, which is publicly available to read in over 20 languages.
- Research Data Alliance (RDA) – an international organization whose main objective is to provide comprehensive information and support regarding the opening and management of research data. The RDA website provides information and recommendations to help in discovering the most appropriate data management solutions. On the site, you can search for information by specific topic or by research area.
- Digital Curation Centre (DCC) – it provides a range of data management information, guides and tools.
- Data Observation Network for Earth (DataONE) – an organization that seeks to ensure open, continuous and secure access to Earth observation research data. It also provides comprehensive methodological material on data management topics.
- CESSDA – Consortium of European Social Science Data Archives, which provides extensive training (in video format, downloadable) on data management topics in the context of Social Sciences, and a Data Management Expert Guide.
-
- Page administrators:
- Jolanta Juršėnė
- Asta Katinaitė-Griežienė
- Olena Dubova
- Orinta Sajetienė
- Ugnė Daraškevičiūtė